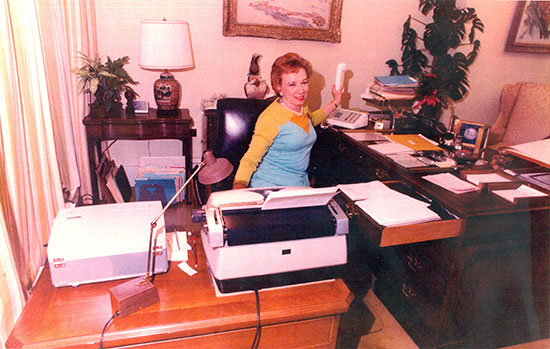
This is an image from the Snowden files. It is labeled “secret.”1Yet one cannot see anything on it.
This is exactly why it is symptomatic.
Not seeing anything intelligible is the new normal. Information is passed on as a set of signals that cannot be picked up by human senses. Contemporary perception is machinic to large degrees. The spectrum of human vision only covers a tiny part of it. Electric charges, radio waves, light pulses encoded by machines for machines are zipping by at slightly subluminal speed. Seeing is superseded by calculating probabilities. Vision loses importance and is replaced by filtering, decrypting, and pattern recognition. Snowden’s image of noise could stand in for a more general human inability to perceive technical signals unless they are processed and translated accordingly.
But noise is not nothing. On the contrary, noise is a huge issue, not only for the NSA but for machinic modes of perception as a whole.
Signal v. Noise was the title of a column on the internal NSA website running from 2011 to 2012. It succinctly frames the NSA’s main problem: how to extract “information from the truckloads of data”:
It’s not about the data or even access to the data. It’s about getting information from the truckloads of data … Developers, please help! We’re drowning (not waving) in a sea of data—with data, data everywhere, but not a drop of information.2
Analysts are choking on intercepted communication. They need to unscramble, filter, decrypt, refine, and process “truckloads of data.” The focus moves from acquisition to discerning, from scarcity to overabundance, from adding on to filtering, from research to pattern recognition. This problem is not restricted to secret services. Even WikiLeaks Julian Assange states: “We are drowning in material.”3
Apophenia
But let’s return to the initial image. The noise on it was actually decrypted by GCHQ technicians to reveal a picture of clouds in the sky. British analysts have been hacking video feeds from Israeli drones at least since 2008, a period which includes the recent IDF aerial campaigns against Gaza.4 But no images of these attacks exist in Snowden’s archive. Instead, there are all sorts of abstract renderings of intercepted broadcasts. Noise. Lines. Color patterns.5 According to leaked training manuals, one needs to apply all sorts of massively secret operations to produce these kinds of images.6
But let me tell you something. I will decrypt this image for you without any secret algorithm. I will use a secret ninja technique instead. And I will even teach you how to do it for free. Please focus very strongly on this image right now.
Doesn’t it look like a shimmering surface of water in the evening sun? Is this perhaps the “sea of data” itself? An overwhelming body of water, which one could drown in? Can you see the waves moving ever so slightly?
I am using a good old method called apophenia.
Apophenia is defined as the perception of patterns within random data.7 The most common examples are people seeing faces in clouds or on the moon. Apophenia is about “drawing connections and conclusions from sources with no direct connection other than their indissoluble perceptual simultaneity,” as Benjamin Bratton recently argued.8
One has to assume that sometimes, analysts also use apophenia.
Someone must have seen the face of Amani al-Nasasra in a cloud. The forty-three-year-old was blinded by an aerial strike in Gaza in 2012 in front of her TV:
“We were in the house watching the news on TV. My husband said he wanted to go to sleep, but I wanted to stay up and watch Al Jazeera to see if there was any news of a ceasefire. The last thing I remember, my husband asked if I changed the channel and I said yes. I didn’t feel anything when the bomb hit—I was unconscious. I didn’t wake up again until I was in the ambulance.” Amani suffered second degree burns and was largely blinded.9
What kind of “signal” was extracted from what kind of “noise” to suggest that al-Nasasra was a legitimate target? Which faces appear on which screens, and why? Or to put it differently: Who is “signal,” and who disposable “noise”?
Pattern Recognition
Jacques Rancière tells a mythical story about how the separation of signal and noise might have been accomplished in Ancient Greece. Sounds produced by affluent male locals were defined as speech, whereas women, children, slaves, and foreigners were assumed to produce garbled noise.10 The distinction between speech and noise served as a kind of political spam filter. Those identified as speaking were labeled citizens and the rest as irrelevant, irrational, and potentially dangerous nuisances. Similarly, today, the question of separating signal and noise has a fundamental political dimension. Pattern recognition resonates with the wider question of political recognition. Who is recognized on a political level and as what? As a subject? A person? A legitimate category of the population? Or perhaps as “dirty data”?
What are dirty data? Here is one example:
Sullivan, from Booz Allen, gave the example the time his team was analyzing demographic information about customers for a luxury hotel chain and came across data showing that teens from a wealthy Middle Eastern country were frequent guests.
“There were a whole group of 17 year-olds staying at the properties worldwide,” Sullivan said. “We thought, ‘That can’t be true.’”11
The demographic finding was dismissed as dirty data—a messed up and worthless set of information—before someone found out that, actually, it was true.
Brown teenagers, in this worldview, are likely to exist. Dead brown teenagers? Why not? But rich brown teenagers? This is so improbable that they must be dirty data and cleansed from your system! The pattern emerging from this operation to separate noise and signal is not very different from Rancière’s political noise filter for allocating citizenship, rationality, and privilege. Affluent brown teenagers seem just as unlikely as speaking slaves and women in the Greek polis.
On the other hand, dirty data are also something like a cache of surreptitious refusal; they express a refusal to be counted and measured:
A study of more than 2,400 UK consumers by research company Verve found that 60% intentionally provided wrong information when submitting personal details online. Almost one quarter (23 percent) said they sometimes gave out incorrect dates of birth, for example, while 9 percent said they did this most of the time and 5 percent always did it.12
Dirty data is where all of our refusals to fill out the constant onslaught of online forms accumulate. Everyone is lying all the time, whenever possible, or at least cutting corners. Not surprisingly, the “dirtiest” area of data collection is consistently pointed out to be the health sector, especially in the US. Doctors and nurses are singled out for filling out forms incorrectly. It seems that health professionals are just as unenthusiastic about filling out forms for systems designed to replace them, as consumers are about performing clerical work for corporations that will spam them in return.
In his book The Utopia of Rules, David Graeber gives a profoundly moving example of the forced extraction of data. After his mom suffered a stroke, he went through the ordeal of having to apply for Medicaid on her behalf:
I had to spend over a month … dealing with the ramifying consequences of the act of whatever anonymous functionary in the New York Department of Motor Vehicles had inscribed my given name as “Daid,” not to mention the Verizon clerk who spelled my surname “Grueber.” Bureaucracies public and private appear—for whatever historical reasons—to be organized in such a way as to guarantee that a significant proportion of actors will not be able to perform their tasks as expected.13
Graeber goes on to call this an example of utopian thinking. Bureaucracy is based on utopian thinking because it assumes people to be perfect from it’s own point of view. Graeber’s mother died before she was accepted into the program.
The endless labor of filling out completely meaningless forms is a new kind of domestic labor in the sense that it is not considered labor at all and assumed to be provided “voluntarily” or performed by underpaid so-called data janitors.14 Yet all the seemingly swift and invisible action of algorithms, their elegant optimization of everything, their recognition of patterns and anomalies—this is based on the endless and utterly senseless labor of providing or fixing messy data.
Dirty data is simply real data in the sense that it documents the struggle of real people with a bureaucracy that exploits the uneven distribution and implementation of digital technology.15 Consider the situation at LaGeSo (the Health and Social Affairs Office) in Berlin, where refugees are risking their health on a daily basis by standing in line outdoors in severe winter weather for hours or even days just to have their data registered and get access to services to which they are entitled (for example, money to buy food).16 These people are perceived as anomalies because, in addition to having the audacity to arrive in the first place, they ask that their rights be respected. There is a similar political algorithm at work: people are blanked out. They cannot even get to the stage to be recognized as claimants. They are not taken into account.
On the other hand, technology also promises to separate different categories of refugees. IBM’s Watson AI system was experimentally programmed to potentially identify terrorists posing as refugees:
IBM hoped to show that the i2 EIA could separate the sheep from the wolves: that is, the masses of harmless asylum-seekers from the few who might be connected to jihadism or who were simply lying about their identities …
IBM created a hypothetical scenario, bringing together several data sources to match against a fictional list of passport-carrying refugees. Perhaps the most important dataset was a list of names of casualties from the conflict gleaned from open press reports and other sources. Some of the material came from the Dark Web, data related to the black market for passports; IBM says that they anonymized or obscured personally identifiable information in this set …
Borene said the system could provide a score to indicate the likelihood that a hypothetical asylum seeker was who they said they were, and do it fast enough to be useful to a border guard or policeman walking a beat.17
The cross-referencing of unofficial databases, including dark web sources, is used to produce a “score,” which calculates the probability that a refugee might be a terrorist. The hope is for a pattern to emerge across different datasets, without actually checking how or if they correspond to any empirical reality. This example is actually part of a much larger subset of “scores”: credit scores, academic ranking scores, scores ranking interaction on online forums etc., which classify people according to financial interactions, online behavior, market data, and other sources. A variety of inputs are boiled down to a single number—a superpattern—which may be a “threat” score or a “social sincerity score,” as planned by Chinese authorities for every single citizen within the next decade. But the input parameters are far from being transparent or verifiable. And while it may be seriously desirable to identify Daesh moles posing as refugees, a similar system seems to have worrying flaws.
The NSA’s SKYNET program was trained to find terrorists in Pakistan by sifting through cell phone customer metadata. But experts criticize the NSA’s methodologies. “There are very few ‘known terrorists’ to use to train and test the model,” explained Patrick Ball, a data scientist and director of the Human Rights Data Analysis Group, to Ars Technica. “If they are using the same records to train the model as they are using to test the model, their assessment of the fit is completely bullshit.”18
Human Rights Data Analysis Group estimates that around 99,000 Pakistanis might have ended up wrongly classified as terrorists by SKYNET, a statistical margin of error that might have had deadly consequences given the fact that the US is waging a drone war on suspected militants in the country and between 2500 and four thousand people are estimated to have been killed since 2004: “In the years that have followed, thousands of innocent people in Pakistan may have been mislabelled as terrorists by that ‘scientifically unsound’ algorithm, possibly resulting in their untimely demise.”19
One needs to emphasize strongly that SKYNET’s operations cannot be objectively assessed, since it is not known how it’s results were utilized. It was most certainly not the only factor in determining drone targets.20 But the example of SKYNET demonstrates just as strongly that a “signal” extracted by assessing correlations and probabilities is not the same as an actual fact, but determined by the inputs the software uses to learn, and the parameters for filtering, correlating, and “identifying.” The old engineer wisdom “crap in—crap out” seems to still apply. In all of these cases—as completely different as they are technologically, geographically, and also ethically—some version of pattern recognition was used to classify groups of people according to political and social parameters. Sometimes it is as simple as, we try to avoid registering refugees. Sometimes there is more mathematical mumbo jumbo involved. But many methods used are opaque, partly biased, exclusive, and—as one expert points out—sometimes also “ridiculously optimistic.”21
Corporate Animism
How to recognize something in sheer noise? A striking visual example of pure and conscious apophenia was recently demonstrated by research labs at Google:22
We train an artificial neural network by showing it millions of training examples and gradually adjusting the network parameters until it gives the classifications we want. The network typically consists of 10–30 stacked layers of artificial neurons. Each image is fed into the input layer, which then talks to the next layer, until eventually the “output” layer is reached. The network’s “answer” comes from this final output layer.23
Neural networks were trained to discern edges, shapes, and a number of objects and animals and then applied to pure noise. They ended up “recognizing” a rainbow-colored mess of disembodied fractal eyes, mostly without lids, incessantly surveilling their audience in a strident display of conscious pattern overidentification.
Google researchers call the act of creating a pattern or an image from nothing but noise “inceptionism” or “deep dreaming.” But these entities are far from mere hallucinations. If they are dreams, those dreams can be interpreted as condensations or displacements of the current technological disposition. They reveal the networked operations of computational image creation, certain presets of machinic vision, its hardwired ideologies and preferences.
One way to visualize what goes on is to turn the network upside down and ask it to enhance an input image in such a way as to elicit a particular interpretation. Say you want to know what sort of image would result in “Banana.” Start with an image full of random noise, then gradually tweak the image towards what the neural net considers a banana. By itself, that doesn’t work very well, but it does if we impose a prior constraint that the image should have similar statistics to natural images, such as neighboring pixels needing to be correlated.24
In a feat of genius, inceptionism manages to visualize the unconscious of prosumer networks: images surveilling users, constantly registering their eye movements, behavior, preferences, aesthetically helplessly adrift between Hundertwasser mug knockoffs and Art Deco friezes gone ballistic. Walter Benjamin’s “optical unconscious” has been upgraded to the unconscious of computational image divination.25
By “recognizing” things and patterns that were not given, inceptionist neural networks eventually end up effectively identifying a new totality of aesthetic and social relations. Presets and stereotypes are applied, regardless of whether they “apply” or not: “The results are intriguing—even a relatively simple neural network can be used to over-interpret an image, just like as children we enjoyed watching clouds and interpreting the random shapes.”26
But inceptionism is not just a digital hallucination. It is a document of an era that trains smartphones to identify kittens, thus hardwiring truly terrifying jargons of cutesy into the means of production.27 It demonstrates a version of corporate animism in which commodities are not only fetishes but morph into franchised chimeras.
Yet these are deeply realist representations. According to György Lukacs, “classical realism” creates “typical characters,” insofar as they represent the objective social (and in this case technological) forces of our times.28
Inceptionism does that and more. It also gives those forces a face—or more precisely, innumerable eyes. The creature that stares at you from your plate of spaghetti and meatballs is not an amphibian beagle. It is the ubiquitous surveillance of networked image production, a form of memetically modified intelligence that watches you in the shape of the lunch that you will Instagram in a second if it doesn’t attack you first. Imagine a world of enslaved objects remorsefully scrutinizing you. Your car, your yacht, your art collection observes you with a gloomy and utterly desperate expression. You may own us, they seem to say, but we are going to inform on you. And guess what kind of creature we are going to recognize in you!29
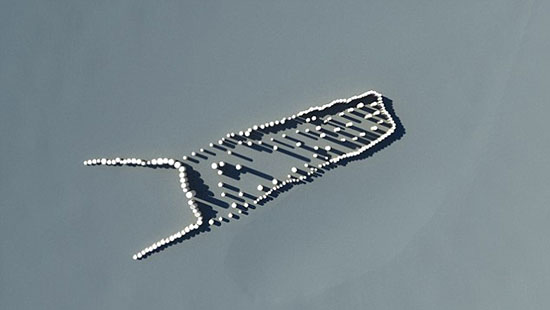
Data Neolithic
But what are we going to make of automated apophenia?30 Are we to assume that machinic perception has entered its own phase of magical thinking? Is this what commodity enchantment means nowadays: hallucinating products? It might be more accurate to assume that humanity has entered yet another new phase of magical thinking. The vocabulary deployed for separating signal and noise is surprisingly pastoral: data “farming” and “harvesting,” “mining” and “extraction” are embraced as if we lived through another massive neolithic revolution31 with it’s own kind of magic formulas.
All sorts of agricultural and mining technologies—that were developed during the neolithic—are reinvented to apply to data. The stones and ores of the past are replaced by silicone and rare earth minerals, while a Minecraft paradigm of extraction describes the processing of minerals into elements of information architecture.32
Pattern recognition was an important asset of neolithic technologies too. It marked the transition between magic and more empirical modes of thinking. The development of the calendar by observing patterns in time enabled more efficient irrigation and agricultural scheduling. Storage of cereals created the idea of property. This period also kick-started institutionalized religion and bureaucracy, as well as managerial techniques including laws and registers. All these innovations also impacted society: hunter and gatherer bands were replaced by farmer kings and slaveholders. The neolithic revolution was not only technological but also had major social consequences.
Today, expressions of life as reflected in data trails become a farmable, harvestable, minable resource managed by informational biopolitics.33
And if you doubt that this is another age of magical thinking, just look at the NSA training manual for unscrambling hacked drone intercepts. As you can see, you need to bewitch the files with a magic wand. (Image Magick is a free image converter):
The supposedly new forms of governance emerging from these technologies look partly archaic and partly superstitious. What kind of corporate/state entities are based on data storage, image unscrambling, high-frequency trading, and Daesh Forex gaming? What are the contemporary equivalents of farmer kings and slaveholders, and how are existing social hierarchies radicalized through examples as vastly different as tech-related gentrification and jihadi online forum gamification? How does the world of pattern recognition and big-data divination relate to the contemporary jumble of oligocracies, troll farms, mercenary hackers, and data robber barons supporting and enabling bot governance, Khelifah clickbait and polymorphous proxy warfare? Is the state in the age of Deep Mind, Deep Learning, and Deep Dreaming a Deep State™? One in which there is no appeal nor due process against algorithmic decrees and divination?
But there is another difference between the original and the current type of “neolithic,” and it harks back to pattern recognition. In ancient astronomy, star constellations were imagined by projecting animal shapes into the skies. After cosmic rhythms and trajectories had been recorded on clay tablets, patterns of movement started to emerge. As additional points of orientation, some star groups were likened to animals and heavenly beings. However, progress in astronomy and mathematics happened not because people kept believing there were animals or gods in space, but on the contrary, because they accepted that constellations were expressions of a physical logic. The patterns were projections, not reality. While today statisticians and other experts routinely acknowledge that their findings are mostly probabilistic projections, policymakers of all sorts conveniently ignore this message. In practice you become coextensive with the data-constellation you project. Social scores of all different kinds—credit scores, academic scores, threat scores—as well as commercial and military pattern-of-life observations impact the real lives of real people, both reformatting and radicalizing social hierarchies by ranking, filtering, and classifying.
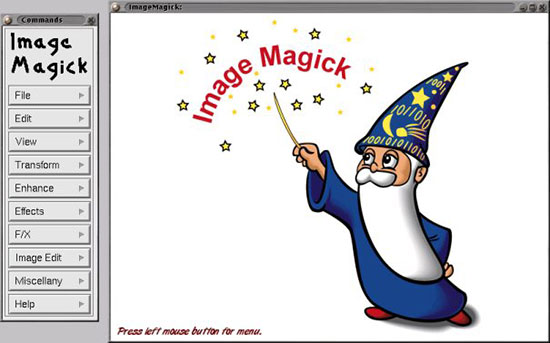
Gestalt Realism
But let’s assume we are actually dealing with projections. Once one accepts that the patterns derived from machinic sensing are not the same as reality, information definitely becomes available with a certain degree of veracity.
Let’s come back to Amani al-Nasasra, the woman blinded by an aerial attack in Gaza. We know: the abstract images recorded as intercepts of IDF drones by British spies do notshow the aerial strike in Gaza that blinded her in 2012. The dates don’t match. There is noevidence in Snowden’s archive. There are no images of this attack, at least as far as I know of. All we know is what she told Human Rights Watch. This is what she said: “I can’t see—ever since the bombing, I can only see shadows.”34
So there is one more way to decode this image. It’s plain for everyone to see. We see what Amani cannot see.
In this case, the noise must be a “document” of what she “sees” now: “the shadows.”
Is this a document of the drone war’s optical unconscious? Of it’s dubious and classified methods of “pattern recognition”? And if so, is there a way to ever “unscramble” the “shadows” Amani has been left with?
×
Acknowledgments: The initial version of this text was written at the request of Laura Poitras, who most generously allowed access to some unclassified documents from the Snowden archive, and a short version was presented during the opening of her show “Astro Noise” at the Whitney Museum. Further thanks to Henrik Moltke for facilitating access to the documents, to Brenda and other members of Laura’s studio, to Linda Stupart for introducing me to the term “apophenia,” and to Ben Bratton for fleshing it out for me.
© 2016 e-flux and the author